- University of Kent
- Kent Business School
- People
- Dr Lina Simeonova
Dr Lina Simeonova
Director of Studies
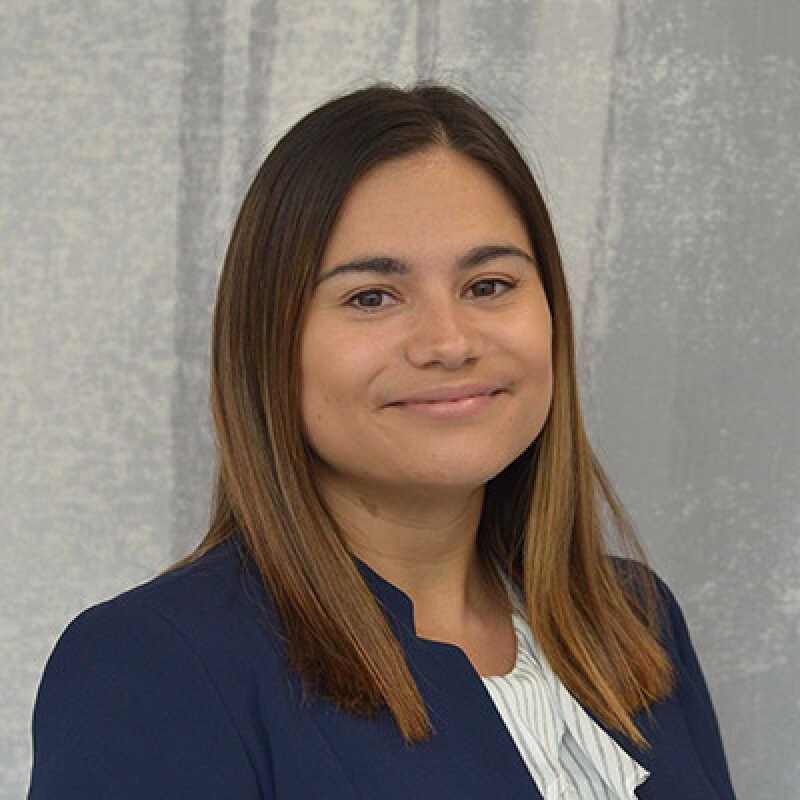
Lina Simeonova is a Lecturer in Operations Management at Kent Business School. She was awarded a PhD in Management Science in 2016 from the University of Kent. Previously she has worked as a Project Manager and Researcher on a Knowledge Transfer Partnership, where she worked towards bridging the gap between industry and academia by successfully implementing Operational Research techniques in real settings.
She was a lead researcher for Kent Police Headquarters on a large-scale multinational project for fighting online crime, focusing on the development of State-of-the-Art algorithms for crime prevention, which were disseminated internationally. She has participated in various events across Kent aimed at encouraging and preparing young students to enrol into higher education.
Lina’s research is mainly in the area of Management Science and Operations Research, with a focus on routing and scheduling, and exploring the benefits of integrated operations in logistics and supply chain.
She finds inspiration for her research from real-life operations and aims to research problems, which are practically applicable and have a positive impact on businesses, society and the environment.
Lina has teaching experience on all academic levels, from primary school to Master’s level in a variety of subjects, including Operations Management, Supply Chain, Spreadsheet Modelling, Data Mining and Forecasting.
Her aim is to deliver all lectures in an academically informative manner, but also to show the relevance of the teaching material in an industry context.
Lina has provided real-life projects and co-supervised dissertations of MSc students for a number of years.
Lina is certified in a number of professional qualifications, including Prince2 Project Management, Agile Project Management, SQL Databases and holds a Green Belt for Lean Six Sigma.
Loading publications...
Showing of total publications in the Kent Academic Repository. View all publications