- University of Kent
- School of European Culture and Languages
- People
- Professor Jon Williamson
Professor Jon Williamson
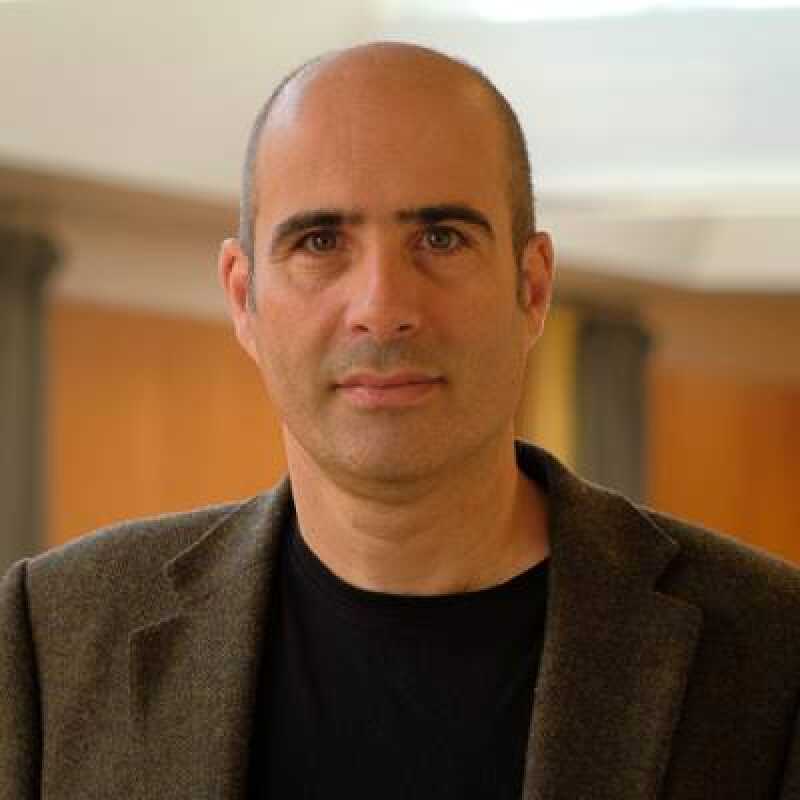
Jon Williamson works in the area of philosophy of science and medicine. He is co-director of the Centre for Reasoning and is a member of the Theoretical Reasoning research cluster.
Professor Jon Williamson works on the philosophy of causality, the foundations of probability, formal epistemology, inductive logic, and the use of causality, probability and inference methods in science and medicine.
His books Bayesian Nets and Causality and In Defence of Objective Bayesianism develop the view that causality and probability are features of the way we reason about the world, not a part of the world itself. His books Probabilistic Logics and Probabilistic Networks and Lectures on Inductive Logic apply recent developments in Bayesianism to motivate a new approach to inductive logic.
Jon's latest book, Evidential Pluralism in the Social Sciences, provides a new account of causal enquiry in the social sciences. His previous book, Evaluating Evidence of Mechanisms in Medicine, seeks to broaden the range of evidence considered by evidence-based medicine. Jon can be found on Twitter @Jon_Williamson_.
Jon teaches logic, reasoning, and philosophy of cognitive science and AI.
Loading publications...
Showing of total publications in the Kent Academic Repository. View all publications